ABSTRACT
given a query vertex q in G, to find as outputall the densely connected subgraphs of G, each of whichcontains the query v.
community detection problem, community search.
In this paper, we study the community searchproblem in the truss-based model aimed at discovering alldense and cohesive k-truss communities to which the query vertex q belongs.
Consequently,all the edges of G can be partitioned to a series of k-truss equivalence classes that constitute a space-ecient, trusspreserving index structure, EquiTruss.
TCP-Index.
1. INTRODUCTION
Given a graph G, a k-truss (k >= 2) is the largest subgraph of G with each constituent edge contained in at least (k - 2) triangles.
modelling real-world community structures, including clique or quasiclique, k-core , k-truss , and nucleus , to name a few.
1.1 choice of k-truss by the following important facts
- (1) as opposed to primitive vertices/edges, the higher-order graph motif, triangle, is exploited as building blocks to quantify the strong and stable relationships in communities .
As a result, the high-density and cohesiveness of real-world
communities can be encoded in k-truss with strong theoretical
guarantees (more details will be elaborated in Section
2.2); - (2) k-truss enables a comprehensive modelling of
multiple, overlapping communities in G. By tuning the parameter
k, we can derive a collection of k-truss communities
that form an inclusive, dense-graph hierarchy representing
the cores of G at varied levels of granularity [26]; - (3) discovering
all k-trusses from G is polynomially tractable [33],
while most existing dense-graph models render the community
search problem NP-hard [13, 6]. As a consequence, ktruss
has been extensively employed for community search in real-world networked applications [12, 14, 13, 11, 33].
a toy graph G
1.2 the concept of k-truss equivalence
given two edges e and e0 of G, they are k-truss equivalent if and only if they belong to the same k-truss, and are further connected by a series of triangles in a strong sense (modeled by the notion of k-triangle connectivity in Definition 9).
2. BACKGROUND
2.1 Preliminaries
Definition 1 - Edge Support(支持度)
denote:sup(e) = k
支持度等于以这条边组成的三角形个数,边中间没有节点。
Definition 2 - Subgraph Trussness
denote:$\tau(G’) = k$
在一个k-子图中每条边的支持度大于等于k-2.
Definition 3 - Triangle Adjacency
denote:${\Delta_1 \bigcap \Delta_2} \neq {\phi} $
两个三角形有公共边,针对于两个三角形。
Definition 4 - Triangle Connectivity
denote:$\Delta_s \leftrightarrow \Delta_t$
这个概念相对于定义3差不多,只是补充说明了如果两个三角形是连接的,但不一定是邻接的,只要中间存在一系列的三角形进行传递,则两个三角形中的任意边可表示为$e \leftrightarrow e’$.
Consider two triangles s and t in G. They are connected, denoted as $e \leftrightarrow e’$.
- 这两条边在同一个三角形里面
- 两个三角形是连接的,则这任意两条边是连接的。$e \leftrightarrow e’$.
They are connected, denoted as $\Delta_s \leftrightarrow \Delta_t$, if there exist a sequence of n triangles $\Delta_1, …, \Delta_n$ in G (n >= 2), such that $\Delta_1 = \Delta_s, \Delta_n = \Delta_t,$ and for 1 <= i < n, $\Deltai \bigcap \Delta{i + 1} \neq \phi $.
翻译过来,这里存在一系列的n个三角形,相邻的两个三角形拥有共享边。
Definition 5 - k-truss Community
- G’ is a k-truss
- $\forall e,e’ \in E_{G’}, e \leftrightarrow e’$
Definition 6 - k-truss community search
find all k-truss communities containing query vertex q.
2.2 Related Work
Community search
Truss
k-core (each vertex in a k-core has its degree no less than k)
Truss is defined on the higher-order graph motif, triangle, and enjoys numerous advantages in community modelling and computation : a k-truss is a (k - 1)-core but not vice versa; a k-truss is (k - 1)-edge-connected: any deletion of fewer than (k - 1) edges will not disconnect a k-truss; a ktruss with n vertices has its diameter no more than $\left \lfloor {\frac{2n - 2}{k}} \right \rfloor$; that is, a k-truss is diameter-bounded.
An improved algorithm was further proposed based on triangle enumeration in O(|EG|^{3/2}) time . A similar I/O-ecient algorithm for k-truss computation was designed and facilitated by graph database technologies . A parallel method, PETA, can detect local k-trusses within a few iterations, and it has the same complexity as corresponding serial algorithms .
Graph Summarization
TCP-Index
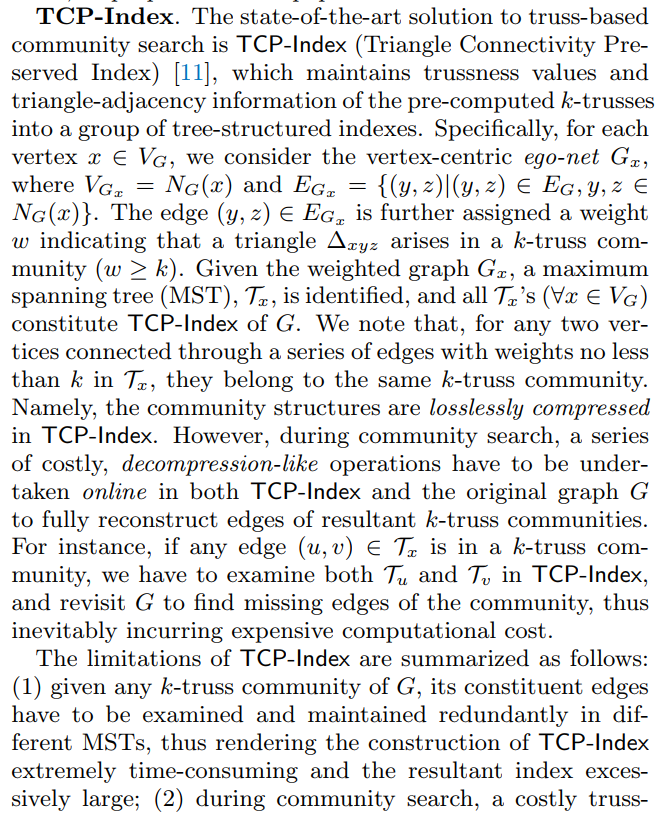
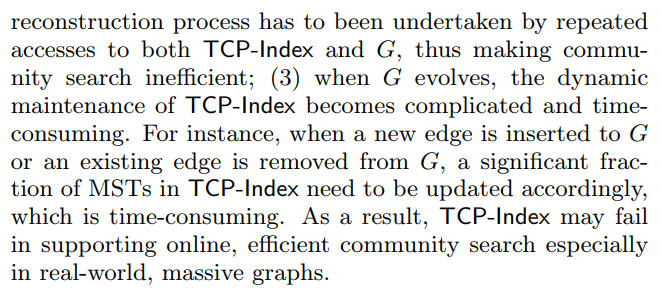
3. TRUSS EQUIVALENCE
Definition 7 - Edge Trussness
denote:$\tau(e) = k$
truss = 支持度 + 2
其实这个我一直认为就是边的支持度。但不能这样认为,这条边的truss值等于边在最大子图中的k-truss中的k值。也就是说
is the maximum subgraph trussness of a subgraph $G \in G$ that involves e as a constituent edge, i.e., $\tau (e) = maxG \subseteq G{\tau (G) : e \in E_{G *}}.$
compute edge supports in O(|E|^1.5)
The time complexity of Algorithm 1 is O(|EG| ^1.5) and its space complexity is O(|VG| + |EG|) .
Definition 8 - k-triangle
三角形中最小的边的truss大于等于k。
Definition 9 - k-triangle connectivity
跟定义8相近。no, no, no。连接是中间可以有一系列的三角形。相邻的三角形的有公共边,并且公共边的truss值为k。强调公共边不能超过k,否则也不算k-triangle connectivity。
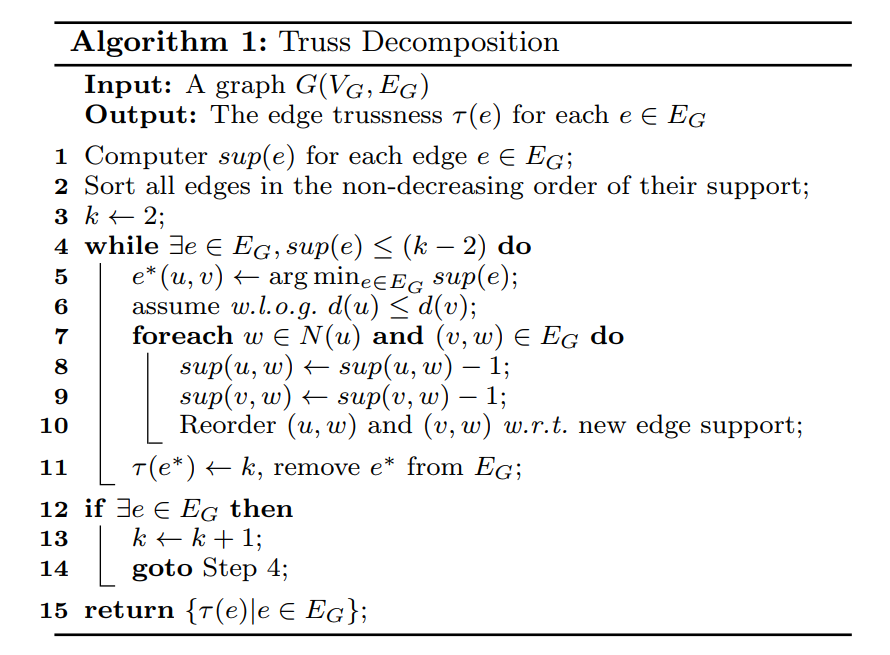
Definition 10 - k-truss equivalence
所有边的truss等于k。
Theorem 1. k-truss equivalence is an equivalence relation upon EG.
4. TRUSS-EQUIVALENCE BASED INDEX
4.1 Index Design and Construction
a graph-structured index, EquiTruss. EquiTruss allows incremental update when G changes dynamically.
- super-node
- super-edge
processed is a Boolean variable indicating whether e has been examined in index construction, and is initialized to FALSE (Line 3); list is a set of super-node identifies, each of which represents a previously explored super-node, µ, where ⌧ (µ) < k, and µ is triangle-connected to the current super-node, ⌫ (⌧ (⌫) = k), via the edge e. The e.list is initialized as an empty set (Line 4).
Theorem 2. EquiTruss can be constructed in O(|EG|^1.5) time and O(|EG|) space by Algorithm 2.
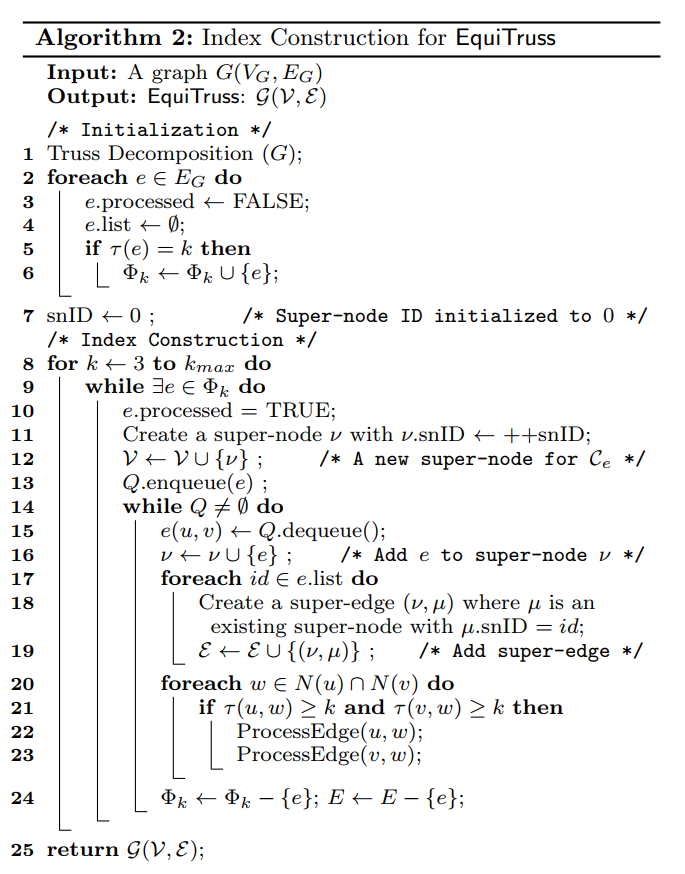
4.2 Community Search on EquiTruss
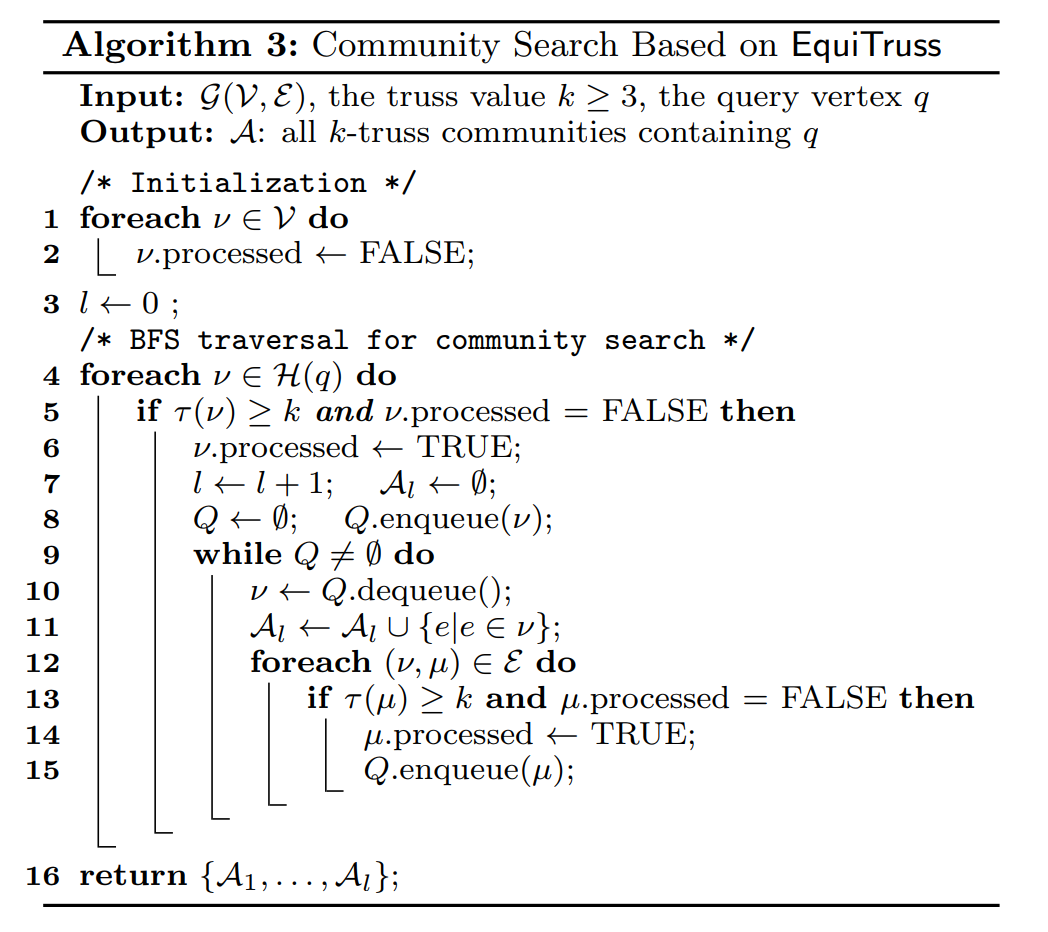
Theorem 3. Given a query vertex q 2 VG and the truss value k, Algorithm 3 correctly computes all k-truss communities containing v.
4.3 Dynamic Maintenance of EquiTruss
未完不待续。。。